A technique to build novel data sets
You're an SDR and you need to find potential customers for your product. Yet you know little about your target market. What should you do? Read: "Everything Starts Out Looking Like a Toy" #190
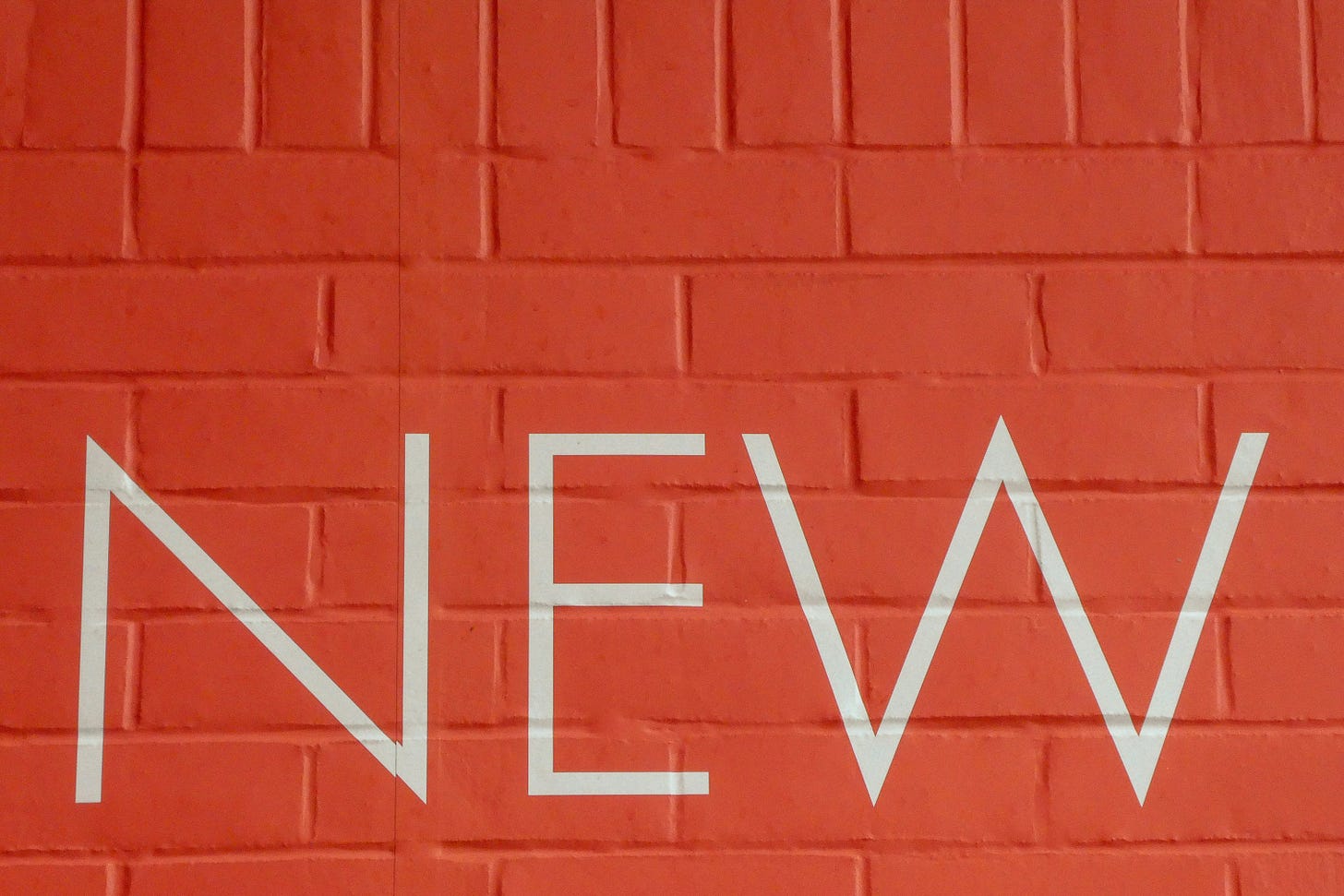
Hi, I’m Greg 👋! I write weekly product essays, including system “handshakes”, the expectations for workflow, and the jobs to be done for data. What is Data Operations? was the first post in the series.
This week’s toy: A newsreel explaining how pictures were sent over wire in the 1930s. This simple idea cleared the way for today’s transmission of … almost anything via the internet. Edition 190 of this newsletter is here - it’s March 18, 2024.
Brought to you by Apollo.io, an all-in-one solution providing RevOps teams with access to data, enrichment, outreach, call intelligence, scoring, calendaring, and email automation. They integrate seamlessly with your CRM and existing workflows. The quality of Apollo’s data is unmatched, they are ranked #1 for contact and company data accuracy on G2 with over 6000 reviews and a 4.8 star rating. Learn more …
If you have a comment or are interested in sponsoring, hit reply.
The Big Idea
A short long-form essay about data things
⚙️ A technique to build novel data sets
When I was building campaigns for OutboundWorks – a company that tried to elevate average SDR behavior to elite levels – one tactic we tried was to automate prospecting steps into groups of steps. We used one set of criteria for building account lists and then overlaid that with contact criteria. The goal was to break down prospecting into something that could be repeated.
The insight we had was that a key issue stopping SDRs from reaching performance targets was a lack of activity cadence and procedure. Breaking each campaign down into a series of steps to build the ideal customer profile (ICP) and another set of steps to identify the right person at that account, we were avoided a lot of the typical challenges of using enrichment data.
For each client, we would find the accounts that match the kind of accounts that were in the ICP, then find look-alikes, then find a systematic way to identify look-alikes, and then find a data source to apply that systematic way and find accounts.
We took a similar approach to contacts by using the words that people describe themselves as in LinkedIn rather than starting from enrichment sources, and then identifying people.
The strategy is not perfect. It works better when contacts create profiles on LinkedIn that are indexed by Google. In recent years as LinkedIn has filled in, this has become a more important information graph even if the specific contact information for individuals isn’t easily available.
Case study: finding buyers at car dealerships
Let’s say you wanted to find parts, managers, and operations, personnel and general managers, and car dealerships in the United States.
If you didn’t have a list of car dealers, you might reverse the search by starting with a list of metropolitan areas by GDP and then building a search in to find car dealerships have known brands.
For typical MSA like Dallas, Texas the search might look like “car dealerships in Dallas Texas” or “Ford dealerships in Dallas Texas”.
Once you find an example and visit their website (let’s say, Planet Ford of Texas), that’s your first hit.
Now search using the LinkedIn parameter and a job title and you’ll get the LinkedIn profiles that Google has indexed for the best match for that role.
In a few steps, we’ve found key contacts, indexed by Google by the suitability for the query (which also tells you how often people search for them relative to other people at that dealership).
Wait, this sounds very much like what you would do when you start your search from inside of Linkedin or by using an enrichment tool.
There’s a key difference.
Why start with a Google Search?
Data enrichment providers are really good at answering your question and giving you back contact information for people and companies you’ve specified. It’s also easy to get a large list of people and not have next steps for filtering them beyond selecting a title or a level - which is very similar to what other SDRs are going to be doing.
By using a novel vector - the search graph provided by Google - you can add the information about popular searches for the type of company or person you’re looking for, indexed by Linkedin.
I used a car dealership here as an example because it’s what you might do if you knew some of the information you wanted to track (there are not that many new car dealer brands, and only some of them have dealerships in each city). If you have other information you’d like to glean (competitors, for example) try a different kind of search.
Often software companies will exist in the space between industry categories and established product areas, especially if they are creating a market. If you are looking for companies that sell continuous delivery software to automate Salesforce you could try to find that hybrid category in account enrichment software. Or you could search “salesforce competitors devops”. Or you could search for novel data:
People in the field who are working on a problem will mention competitors because they are often the benchmark for a problem they are solving. They will probably describe those competitors differently than a product marketing team, leaving clues for elite SDR behavior.
What makes an elite SDR?
Elite sales development representatives are able to unpack the ideal customer profile into signals they can test, like a boolean true/false node. By identifying these pieces and applying them to all of their tools they are able to get to the accounts and people they are looking for faster and more accurately. In addition, they are likely to be fresh and highly accurate signals because they are based on the content people are actively sharing online.
Does this match every selling situation? Of course not. But it’s an excellent backtest to help you understand if the search you are doing for novel data is going to find different answers than other people will typically get for the problem at hand. These answers will help you to frame the benefits of your solution in words the prospect is listening for.
What’s the takeaway? In a world where everyone has similar tools to find and enrich data, how do you make those tools perform much better than other people using that same approach? By adding a vector to validate and find novel information and zero in on the available data more effectively and quickly. You might even find bonus points: the ability to talk to a prospect about something they wrote online instead of just telling them you are a great solution to their problem.
Links for Reading and Sharing
These are links that caught my 👀
1/ Loss aversion is a thing - You have something in your hand. I offer you something else with the same value. Do you take it? Most people don’t. We are biased to believe the thing we have is better than the thing we don’t have, all else being equal. Is this a positive or negative thing? It depends.
2/ Ways to change pricing perception - Speaking of loss aversion, here are a number of ways to change pricing and be more persuasive. After you read this, you’ll view pricing displays quite differently.
3/ On writing - I really appreciate this brief essay on writing. Write because it matters to you - if it matters to you, you’ll find other people who appreciate that work.
What to do next
Hit reply if you’ve got links to share, data stories, or want to say hello.
The next big thing always starts out being dismissed as a “toy.” - Chris Dixon