Bridging the Gap: Uniting Data and Go-to-Market Teams for B2B SaaS Success
The path to product-led growth (PLG) success depends on Data and GTM teams getting along. Read the first in a series: "Everything Starts Out Looking Like a Toy" #144
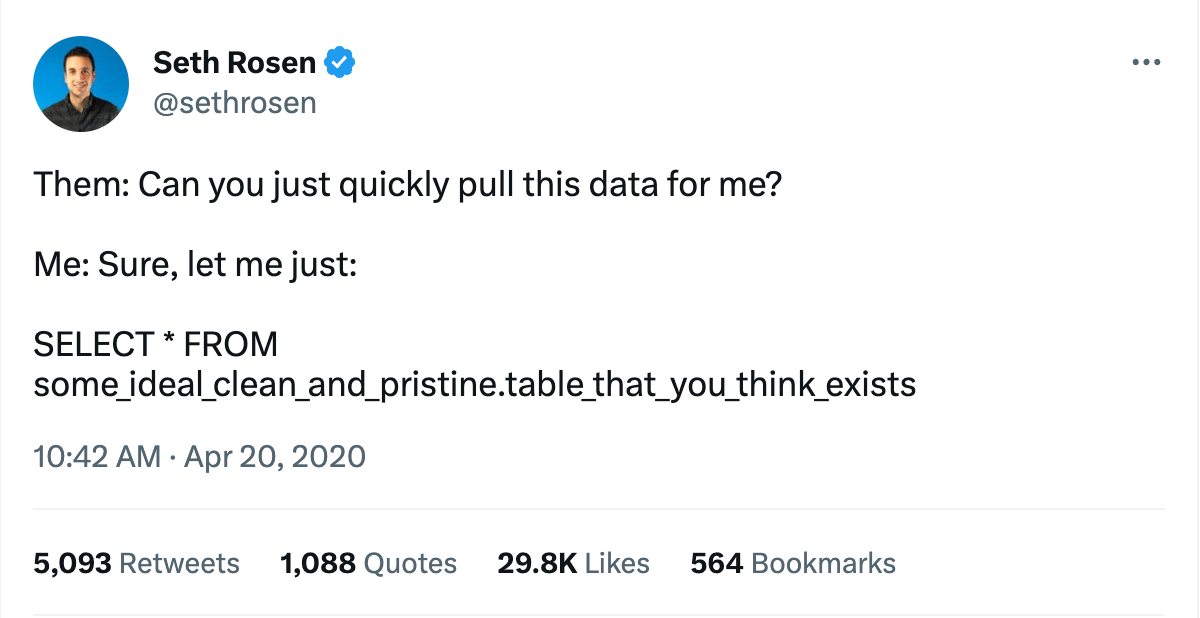
A HUGE thank you to our newsletter sponsor Pocus for your support.
If you're reading this but haven't subscribed, join our community of curious GTM and product leaders. If you’d like to sponsor the newsletter, reply to this email.
Brought to you by Pocus, a Revenue Data Platform built for go-to-market teams to analyze, visualize, and action data about their prospects and customers without needing engineers. Pocus helps companies like Miro, Webflow, Loom, and Superhuman save 10+ hours/week digging through data to surface millions in new revenue opportunities.
Hi, I’m Greg 👋! I write weekly product essays, including system “handshakes”, the expectations for workflow, and the jobs to be done for data. What is Data Operations? A discussion that grew into Data & Ops, a fractional product team.
This week’s toy: a service that summarizes any YouTube video and gives you the tl;dr. It will even generate a thread of Tweets for you - try using it to analyze this video of ChatGPT’s potential to be a super tutor. Edition 144 of this newsletter is here - it’s May 8, 2023.
The Big Idea
A short long-form essay about data things
⚙️ The Path to PLG Success: Data and GTM teams join forces to improve data and next actions
Hello! 👋 Do you ever feel like the communication between data teams and marketing/sales folks in a B2B SaaS environment could use some improvement?
This happens frequently when you are trying to describe an organization-wide process that needs to touch multiple systems, and the teams don’t agree on a key definition or flow. (MQLs, anyone?) It’s a common challenge that can lead to lost revenue and wasted resources, not to mention frustration all around.
If you ask teams, they’re not sure how to phrase this disconnect, and they’d describe it in a few ways:
GTM teams want to define and solve business problems and don’t want to know about the schema of the underlying data
Data teams want to report reliably on data and need confidence that the GTM teams are not proposing changes that will break data schemas
They both need an easy way to know what has happened and what is happening using visualization
The path to product-led growth (PLG) success depends upon these two teams getting along, working together, and collaborating on a solution.
That's why we're excited to launch this blog series. Over the next few posts, we'll be diving into the issues that arise when these teams aren't on the same page and sharing some tips and tricks for building stronger, more collaborative relationships.
Our hope is to inspire a future where data teams and marketing/sales folks work together seamlessly, using data to inform their strategies and drive growth for their companies. We want to empower our readers to take action and make positive changes in their own organizations.
So, join us on this journey as we explore the challenges, share best practices, and envision a brighter future for these critical teams!
Let’s get started.
Bridging the Gap: Uniting Data and Go-to-Market Teams for B2B SaaS Success
The first step to solving a problem is to admit there is a problem and name it.
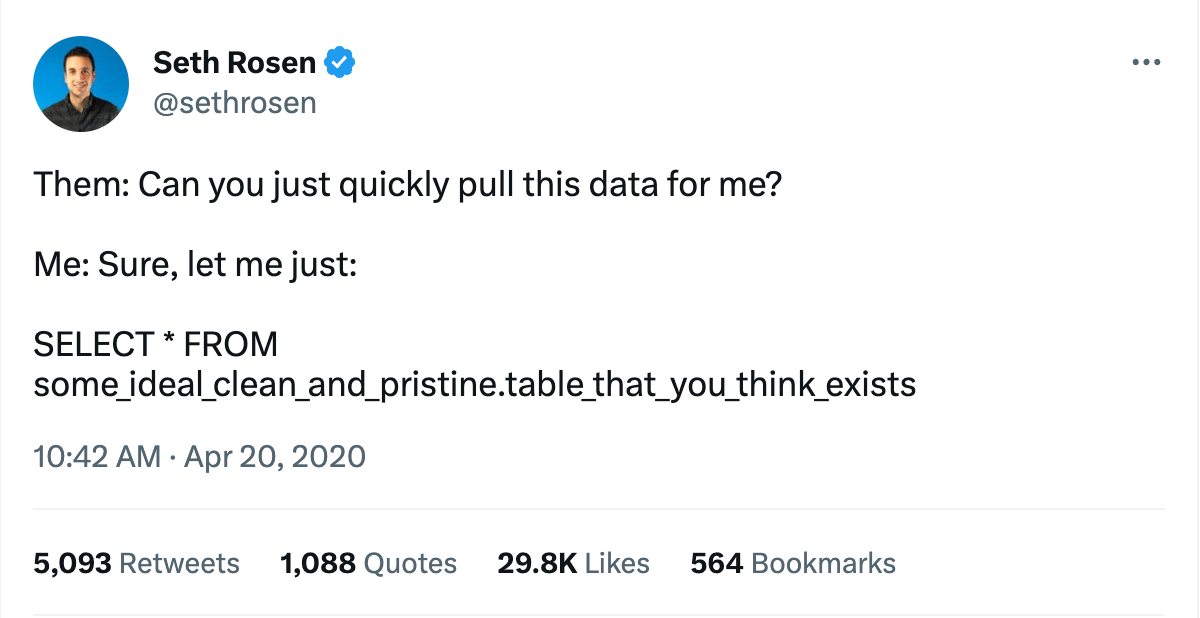
Data teams and Go-to-market (GTM) teams aren’t always focused on the same goals. There always seems to be a shiny object preventing the data team from looking at fixing the important data that is updating the sales pipeline, and the GTM team doesn’t understand the impact of making a seemingly simple request that hides the complexity to be solved by the data team before a “quick question” can be answered. But it can get better.
Here are a few issues that seem to come up all of the time when it comes to Data and GTM teams:
GTM tools are either totally lacking or have incomplete product usage data visibility
Disparate metrics are being created in various tools so there are no standard definitions of something like active user across tools
For companies focused on PLG, the data warehouse usually has a better representation of the full spectrum of business data, but sales teams work out of their own lead/account tools instead of having more relevant and timely information.
Let’s take a look at each one of these to explore how these teams could have better collaboration while achieving shared goals. An aligned team is going to achieve business goals more effectively and efficiently, and knowing you’re after the same goal as your colleague helps the work to be more of a work challenge and less of a personal one.
GTM Tools have incomplete product data
GTM tools are focused on selling to and marketing to prospects. That means their core data set is based on leads, opportunities, and campaigns. A well-orchestrated GTM team will coordinate the data that flows from an initial campaign contact through to a converted lead and subsequent opportunity. CRMs do a great job of typing leads, opportunities, and contacts to an account. But it’s easy for product data for a lead to be out of sync with aggregated product data for that account.
When a lead takes an action in the product, how long does it take to:
Match the data to an account
Identify the type of action
Find the number of times that action has happened in that account
Increment the counter
For most companies, this happens only a few times a day, or as infrequently as daily. That means that for any given product action by a lead, there might be hours of lag before that account has updated product information for that lead/contact.
Why don’t these tools have complete product data? There are a few main reasons:
Product data (especially event data) has a large number of records because it is sampling activity at a high rate. Many GTM tools base their pricing on record count or the size of the database, so adding more records sounds scary.
Applications that store activity data don’t always have clean or easy integrations with CRM applications, and you need a third-party tool to get the data to arrive there
Product data is tied to a person, not aggregated to an eventual account, and there needs to be a process to roll up that data and type it with the meaning of that product data.
All of this means that product signals – the leading indicators of whether someone is engaging with your product in a meaningful way – are not making their way into the selling process. This is important because knowing if someone is engaging during a trial period or if their overall usage has changed significantly is important data to inform sales outreach and the overall buying cycle.
If you don’t have product data, you’re not engaging with the customer where they are. What if you could bring the information from product engagement into the sales cycle to prioritize those prospects who are actually using the product? That’s the essence of PLG: finding the needle in the haystack signals that help you understand the next best action to take.
Metrics do not have a standard definition
That leads to the next disconnect when you think about how to measure the impact of the product data that you’ve discovered. Is it good that someone has logged into the product three times in the last week? Well, any solid engineer would tell you “it depends”. You need the definition of “active user” defined before you can set a reliable threshold of positive or negative activity.
For example, if a data team is using dbt to define the data set for users, you might need to join your users table with an activity table that logs significant activity (logins, actions, and other activity). There is likely an automated job that counts and rolls up this activity into daily sessions and other counts several times a day. And there is another process that selects an audience from this combined user view depending upon that count and whether it exceeds the threshold for an “active user.”
In this case, what’s an “Active user”? It could be any one of these things:
Any user who shows up with activity daily
A customer who takes action every few days
A new user who takes a significant action, for example, the first time they add a new activity.
All of these definitions could be answers to the “active user question” and involve source tables and final models that might not be obvious to the GTM team but represent upstream decisions that need to be made for data management and transformation.
To answer this question, you need one place to go where everyone in the organization can agree on a definition for that metric. You don’t need a “metrics catalog” yet, but even a spreadsheet or a database table showing the name of a thing, its definition, how often it is captured, a high threshold value, a low threshold value, and instructions on what to do if either of those is breached is a great starting point.
This amount of information will tell the GTM and Data teams that were squabbling earlier over the definition of a marketing-qualified lead (MQL):
What it is
Where to find it
When you should pay attention to this value
What you should do when it changes above and below a threshold
Practically speaking, the information is assembled from multiple back-end sources into a transformed view that demonstrates the agreed-upon metric. This is how the process appears in dbt, a popular method of loading, transforming, and delivering data.
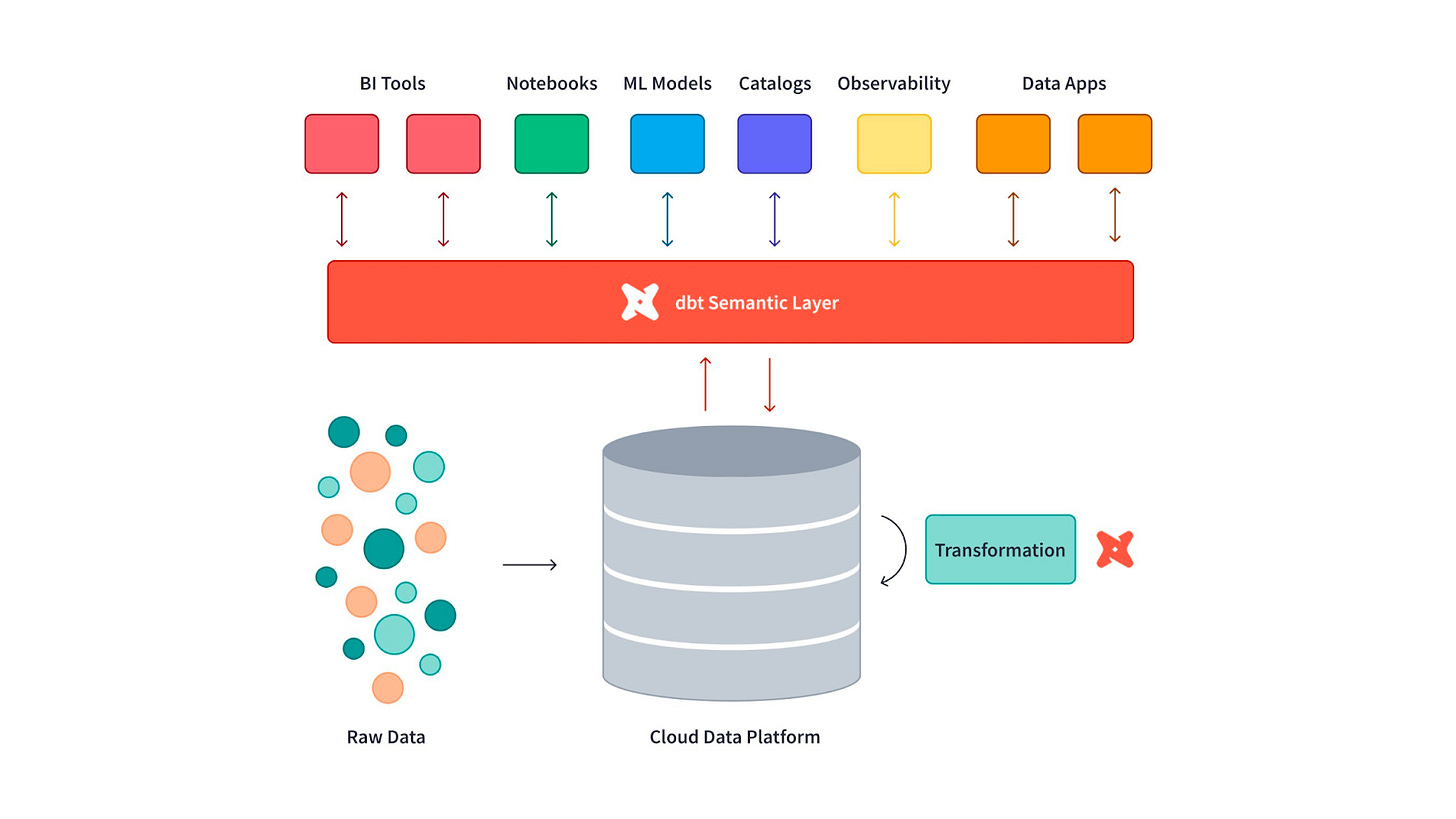
The end metrics (like our definition for MQL) end up in a view we can query from the cloud data platform (Snowflake, Postgres, or similar) but the definitions need to be applied upstream for the data team to be able to understand the metric as a query and to apply it to data sources, tables, and views.
This information gives teams the power to work together to address a problem using data as the measurement and justification for solving the problem. That’s much better than fighting over a definition.
But where do you find the actual answer to your query? It turns out that another problem with the GTM and Data Teams is staring you in the face: the problem that the data in the GTM tools is starting to smell.
Your Warehouse has fresher data than your GTM tools
Your GTM tools have a physics problem. They cannot magically update themselves with the latest product data because their APIs are not built to handle product data. The best solution you have is to take the unique identifier for a person or an account and associate that information with that data in your data warehouse. This presents a problem when an event happens in the product, the information is updated in near-real time in your warehouse, and the GTM tools are struggling to keep up.
What’s a GTM operator to do? One thing to remember is that your warehouse does have fresh data - it just needs to know what are the conditions to take that data and hydrate your account and contact records with important information. Updating things every time someone takes action might be quite noisy and hard to understand, so one of the important tasks, when you establish a metrics catalog, is to think about the key events (or aggregated events) that require action by a human or a process.
Here’s an example of a roll-up field (a count of aggregated events) that might not seem obviously difficult When you count how many “hand raiser” events happened at a company during a day or a week, this means you need to review captured events and filter them for events that meet the criteria of a hand raiser (demo request, new account, or similar).
Then, you need to match the ID of the user in your app with the ID of the user in the CRM, handling any cases where you need to find a missing user, create a new one, or merge existing users as needed to account for multiple emails from the same user. Another example of this challenge is when you roll up domains and workspaces that belong to the same account but might be associated with multiple opportunities. You need to be careful to alert the right rep.
If you know that the data you need can be combined and recombined and transformed, it’s easier to think of it as a product to be delivered to the GTM teams as needed. Delivering the right product at the right time to the right place makes your data shine, and gives you a “one in a row” win to help the GTM team gain trust that you are working on the right problem.
Next time in this series, we’ll cover how Data Teams and GTM teams can solve problems collaboratively using a shared data model.
What’s the takeaway? It’s easy to forget that Data Teams and GTM Teams are trying to solve the same goal: to deliver accurate information to sellers and to the prospect in a timely manner that doesn’t slow things down. The solution resides in defining where product data lives and how to hydrate it into other apps; agreeing on how to work together on change control; and documenting shared metrics from the data warehouse into information the rest of the business can use.
Links for Reading and Sharing
These are links that caught my 👀
1/ LLMs and society - The Economist posits some theories about how large language models and AI are going to affect society. The truth is that we don’t really know what’s going to happen with these tools, except that Pandora’s box has been opened. Whether it will be a net negative or net positive for civilization is going to take decades to figure out. For now, we know that change is constant, and is going to accelerate.
2/ Learn this command to survive! - Lost at SQL is a surprisingly engaging game that helps you to learn the basics of querying a database.
The story game, set in a world where you are a submarine commander needing to run commands on your terminal to help the vessel survive, is more fun than it should be. It reminds me of serial action like Buck Rogers, and you might learn something too!
3/ Deep fake images are getting even better - Stability.ai has released its “Deep Floyd” model that creates text-based images much better than previous models have been able to render. I’m both impressed by what it can do and a bit worried to think about how we’re going to detect these types of fakes going forward.
What to do next
Hit reply if you’ve got links to share, data stories, or want to say hello.
Want to book a discovery call to talk about how we can work together?
The next big thing always starts out being dismissed as a “toy.” - Chris Dixon
Great to see another post addressing what I refer to as "the modern data divide" -- https://databeats.community/p/the-data-divide