Redefining the Customer Journey
A snapshot in time doesn't tell you enough about the customer to know what will happen next - you need a time series. Read: "Everything Starts Out Looking Like a Toy" #167
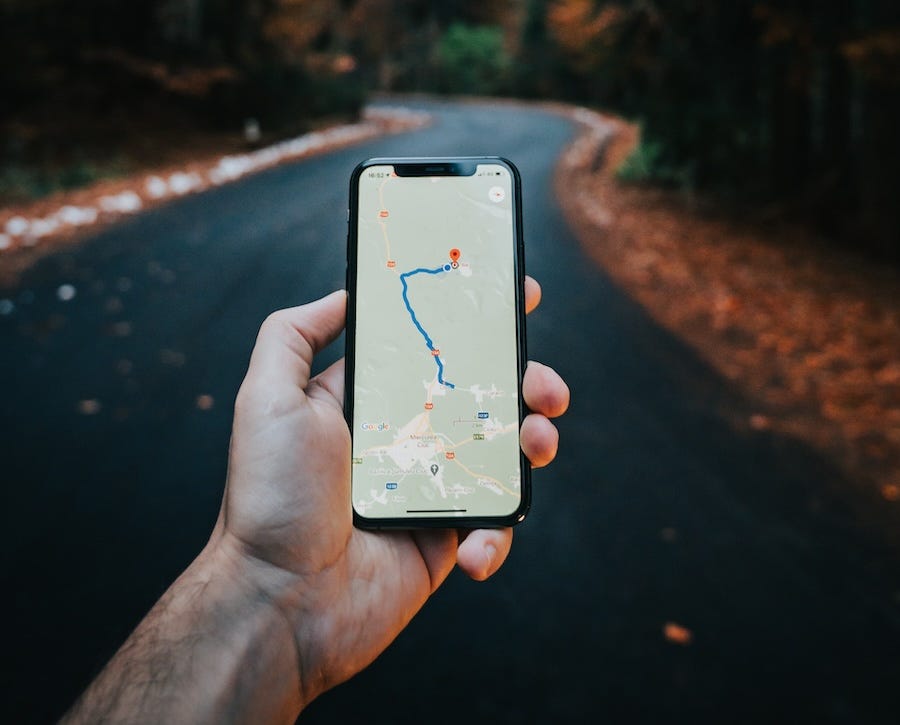
Hi, I’m Greg 👋! I write weekly product essays, including system “handshakes”, the expectations for workflow, and the jobs to be done for data.
This week’s toy: exploratory data analysis on a set of sonnets may seem simple, but gives valuable ideas on evaluating and analyzing any data set. Edition 167 of this newsletter is here - it’s October 16, 2023.
If you have a comment or are interested in sponsoring, hit reply.
The Big Idea
A short long-form essay about data things
⚙️ Redefining the Customer Journey
Have you ever played RevOps detective? 🕵️
The story goes something like this. There’s a closed-won (or a closed-loss) account, and you’re wondering how they got to that point. As you start your investigation, you find that you are missing some key points in the customer’s journey for one reason or another and it’s hard to know what happened. Your Customer Journey Map is incomplete.
What’s in a customer’s journey?
The team at Delighted defines a customer journey map as follows:
A customer journey map is a visual storyline of every engagement a customer has with a service, brand, or product. The customer journey mapping process puts the organization directly in the consumer’s mind to better understand the customer’s processes, needs, and perceptions. (source)
In the B2B world, that journey might look like this:
To create this map, you need to track the prospect from consideration through awareness, activation, and action. Once the prospect becomes a customer, you’ll need to link their prior actions with their customer actions.
In theory, this seems straightforward. When you play detective, you want to go back in time and determine what milestones the customer crossed, when they took those actions, and get a general sense of why they ended up lost or won.
It’s not usually that easy when you find yourself playing detective.
Why don’t more teams focus on the customer journey?
What would a data detective need to solve this problem more effectively? After the fact, it’s not easy to find out what happened for a few reasons:
historical data is almost certainly different than the current state, but the intermediate steps and times might not be captured
the granularity of the information might be different than what you need (you wanted daily data, and you have weekly or monthly data)
it’s not clear when significant milestones happened in the customer journey, particularly if your definitions for those milestones change over time
It’s hard to know what happened, when it happened, and how to relate the things that happened into a timeline that’s easy to consume. To make that journey map, you’ll need to arrange your information in these areas.
Events: What Happened
Data detectives want to know how people arrived at your site, what they did after arriving, and what they did after signing up. How granular should this be? You probably need to balance between capturing an event every time a customer scrolls a menu (though recording their session makes sense) and recording the most important things that happen.
A successful list of events tells the story of that customer’s interaction over time. It’s the customer journey map for that individual customer, and it needs to be pretty detailed.
Milestones: When Did Important Things Happen
There are certain events in the journey that are significant. “Signed up for demo.” “Started a subscription.” “Failed a payment.” “Renewed for another term.” “Canceled.”
In a perfect world, these things happen on a timeline where you can review each event, calculate the difference between this event and another one, and learn a bit more about the journey. In many systems, these events are the names of stages. If you don’t track the date stamp of a significant milestone, you’re missing an important piece of information.
A series of events gives the data detective more information to solve the case.
Trends: What groups of things are happening
A successful data detective reviews trends as part of the investigation process. You’ll want to know if this has happened before, and whether you’ve established a new pattern.
Do this by looking at:
what happened and when (a data series of events)
significant things that happened for this customer (the same, but for specialized events)
the paths the customer took to get to the significant event
Paths give you a sense of journey but not velocity. You’ll know what happened and will be hard to suggest what could happen next.
What can you do about this problem?
Prediction is the big gap in the customer journey. (And in the journey map.)
What would it look like to help future data detectives in your organization?
Make sure you are capturing event history, not just significant events
Think about the required data grain for your metrics. If you need hourly metrics, daily data is not going to do it. (You’ll need a time stamp.)
Start thinking about the next most likely action based on the path and how to highlight it in the customer’s record
Bonus points if you are able to store a data series of decisions made by the organization that affect the “important metrics” and how they are calculated. You could use a form of this decision log.
What’s the takeaway? Data detectives use the events that happen in your customer journey to find out what happened. When these events fit into a story that can be replayed and compared with other customers or prospects, you gain valuable trending information for predicting next actions. Without that consistency, data detectives have more work to do.
Links for Reading and Sharing
These are links that caught my 👀
1/ Keep it simple - it’s a good design intent to start by reducing choice.
2/ What’s AI really good for? - Ben Evans asks the question: what sort of products should you be building with AI? The problem is that it will give you an answer to almost any question you ask it. But how do you know whether the answer you got is close to the right one?
3/ A short history of pivot tables - Let’s celebrate the pivot table. If you haven’t used one before, try it to make a quick GROUP BY query easy to complete from any csv file or other flat file.
What to do next
Hit reply if you’ve got links to share, data stories, or want to say hello.
Want to book a discovery call to talk about how we can work together?
The next big thing always starts out being dismissed as a “toy.” - Chris Dixon
I am rather “new” to the subscription and will go through some historical articles but more like these would be insightful.
Thanks for simplifying the whole process in a digestible manner.