Stay ahead: Exponential growth in AI and GPT means you need to change tactics
A new AI or GPT tool comes out almost every day. How can you keep up and avoid having your knowledge plateau on a local maximum? Read: "Everything Starts Out Looking Like a Toy" #141
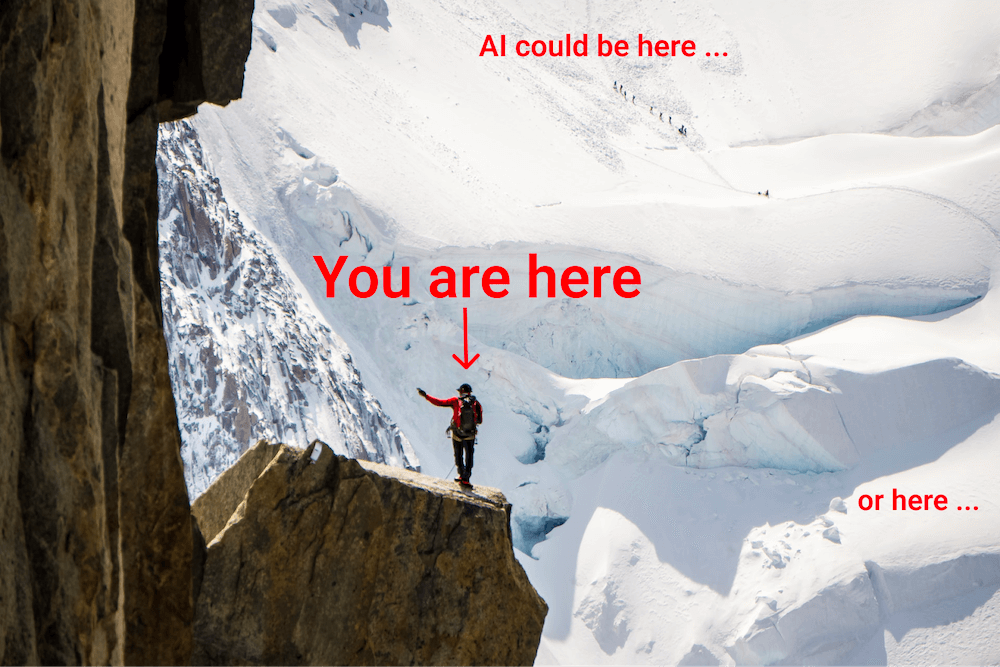
Read Time: 9 minutes
A HUGE thank you to our newsletter sponsor Pocus for your support.
If you're reading this but haven't subscribed, join our community of curious GTM and product leaders. If you’d like to sponsor the newsletter, reply to this email.
Brought to you by Pocus, a Revenue Data Platform built for go-to-market teams to analyze, visualize, and action data about their prospects and customers without needing engineers. Pocus helps companies like Miro, Webflow, Loom, and Superhuman save 10+ hours/week digging through data to surface millions in new revenue opportunities.
Hi, I’m Greg 👋! I write essays on product development, including system “handshakes”, the expectations for workflow, and the jobs we expect data to do. This started with What is Data Operations? It grew into Data & Ops, a fractional product team to create amazing UX and product experiences.
This week’s toy: an AI prompt to create designs inside Figma. One of the things that’s fascinating about compounding technology gains is that simple automation combined with existing processes changes things rapidly. Will this replace a skilled designer? Nope. Should every designer consider using it, if only to build iterations on existing designs? Yes. Edition 141 of this newsletter is here - it’s April 17, 2023.
The Big Idea
A short long-form essay about data things
⚙️ Stay ahead: Exponential growth in AI and GPT means you need to change tactics
We are all on an interesting journey when it comes to learning about AI and GPT technologies. It seems like things are changing fast. Really fast. So fast that it is hard to find the proverbial “you are here” sign on the map that will tell us how we are progressing from what we knew yesterday to what we will know tomorrow to what we might know a few years from now.
This is an inflection point in our understanding of technology. Yet it’s changing at a rate faster than we can comprehend. Consider Moore’s law: the gold standard of technological advancement. If you were standing at the bottom of this curve in 1971, things would look flat or linear. And they have taken off in the last 10 years.
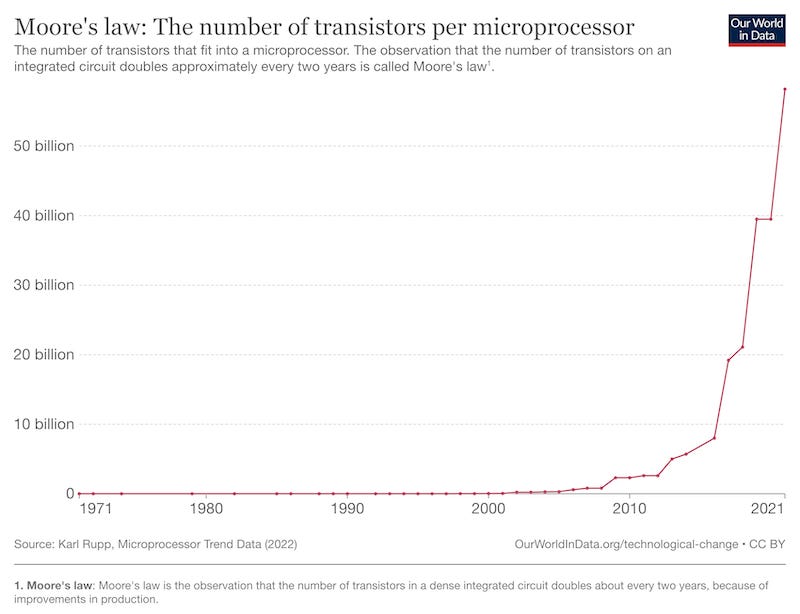
Dealing with our local maximum for understanding
What does Moore’s law mean for the improvement of computer intelligence? We know the “normally accepted” technology limits have changed drastically. Consider the past for inspiration on where AI/GPT is headed.
If we look backward, we see six major technological advances of the last century:
Electricity and electric power distribution - the ability to generate power and move it where we needed it
Telephone - the ability to communicate over long distances without traveling
Automobile - the ability to travel long distances with a personal vehicle
Radio and Television - the ability to communicate to many people simultaneously
Computers and the Internet - the ability to create automated digital workloads and distribute them anywhere
Digital and wireless communication - the ability to access those automated digital workloads from anywhere on Earth
Each of these technological shifts caused big societal changes that altered the lives of everyone on the planet. The second-order effects of these changes compounded as the technology built on itself. Although many negative things happened as a result of these changes, the average person was richer, had more leisure time, and had more access to information. (This puts me out of the AI doomer category, although I’m not an AI enthusiast yet either.)
Machine learning and some sort of artificial intelligence may very well be the next technological paradigm shift in that list. Our local maximum – the point at which our intelligence and comprehension of the technology around us reach a peak value – is not the peak value that technology can achieve.
We just haven’t seen what that looks like yet.
How to deal with AI
What do you do when you encounter a new thing that seems scary and different? I try to learn as much as I can to apply my worldview and know how I need to adapt.
One of the people I often read is Tim O’Reilly (you may know him as the publisher of those programming books with animals on the cover). He’s a veteran technologist and writer who has written the following about understanding how to regulate AI:
These systems are still very much under human control. For now, at least, they do what they are told, and when the results don’t match expectations, their training is quickly improved. What we need to know is what they are being told.
O’Reilly discusses the analog to financial reporting rules, where firms eventually established Generally Accepted Accounting Principles because it benefited everyone involved. Because firms knew how to run their business and customers and prospects knew what to expect, financial reporting became easier to understand.
We are at a similar point in machine learning and AI systems, where we first need to establish the definitions for what’s there. After basic definitions, we need to define the actions that servers and agents can possibly take on data so that we can build use cases and descriptions for the happy path and also for ways we can start to determine when things go wrong. We may even find that the only way to regulate AI is to develop defensive AI that is trained on a different, deliberate set of rules.
Today’s AI looks like it’s thinking, but it isn’t
What is AI actually doing when it is doing its autocompletion thing? Stephen Wolfram writes about this probability machine and how it is using the likelihood of finding the next token in a big list (2048 tokens at the moment, soon to be 8k) compared against the model of about 175 documents. At that point, the “which thing to present next” looks like a math problem to the model.
What could possibly go wrong? This XKCD comic gently points at some of the problems with machine learning when it points in a weird direction. When ChatGPT does this, it’s not actually thinking. It’s suggesting the most likely outcome based on the model. What’s eerie (and triggers the “uncanny valley” response is that the most likely outcome based on the model often resembles the kind of response a person would create.
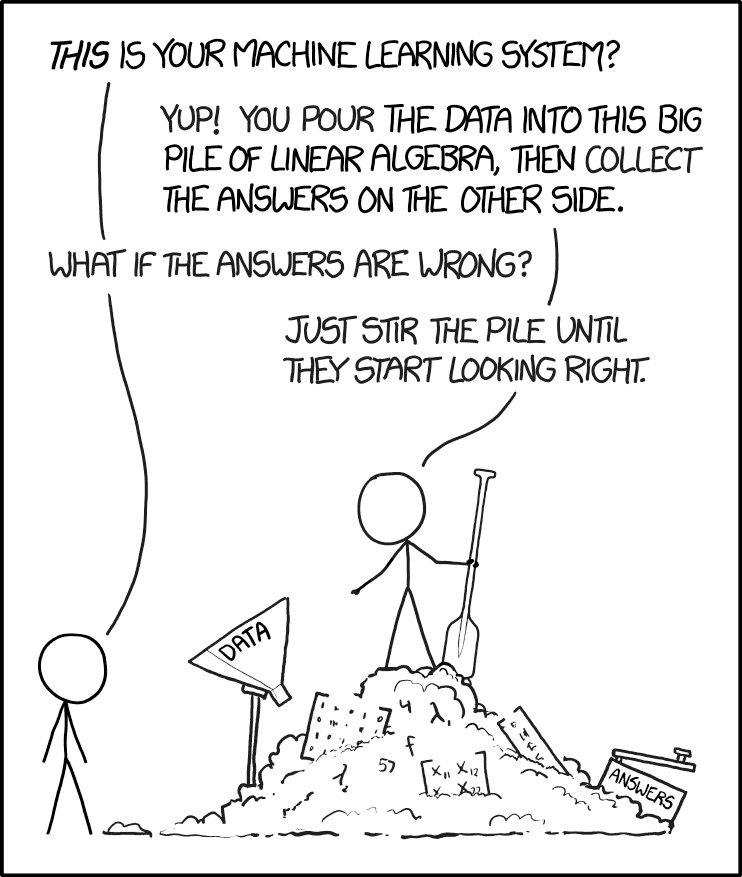
Of course, this leads to humorous responses to prompts, such as this response to Janelle Shane that she writes about when asking ChatGPT to create ASCII art.
ChatGPT can’t do everything we expect yet, and these mistakes will improve quickly. Be aware of what you are seeing, though:
It’s not that the model is suffering errors of perception; it’s attempting to paper over the gaps in a corpus of training data that can’t possibly span every scenario it might encounter.
We need to establish a theory of mind about AI and ChatGPT so that our ethics and personal first principles help us to encounter the various forms of AI in the future.
Tactics to use today to coexist with change
Here are a few things you can do to learn more about AI and GPT and learn where your local maximum of learning lives.
Try talking to an AI tool. They are everywhere these days. You can try something like ChatGPT or use the suggestions in Notion, Google Docs, and more.
Test the limits of what they can do. Like Janelle Shane, try weird and wonderful things to see how they turn out. One place to chat is You.com, and you can test image creation there or at Playground.ai.
Build prompts for these bots or try out samples. FlowGPT is a prompt community where people share examples of prompts.
If you’re more technical, launch a Large Learning Model and host it yourself with Databricks. You can even try this locally or in a browser (you’ll need Chrome 113).
Write a personal code of AI ethics. This doesn’t have to be super complicated – it’s a bullet point list of the things you will and won’t do with AI – and will help you the next time you ask the question of “should you do it?”
This list will not solve all of your problems with AI, and will not make you into an expert. It will give you clues to how you personally want to interact with these tools and more importantly will give you experience and reps in seeing how they respond.
What can companies do to respond to AI? Pay attention to what Tim O’Reilly says:
Companies creating advanced AI should work together to formulate a comprehensive set of operating metrics that can be reported regularly and consistently to regulators and the public, as well as a process for updating those metrics as new best practices emerge.
AI and GPT are just getting started. Building personal tactics to learn more about these technologies will help you to stay up to date. You might learn something too!
What’s the takeaway? The best way to learn more about AI and GPT tools is to try them. Start with something like ChatGPT and then experiment with sequential prompts to explore these capabilities and find something that works for you. Keep in mind things are changing faster than you can respond: the goal is to get familiar with how it feels to use AI.
Links for Reading and Sharing
These are links that caught my 👀
1/ The Lazy Engineer and the Modern Data Stack - the future of the modern data stack looks like more focus on delivering automation, clearer metrics and outcomes, and the concept of “lazy”. Lazy in this case means not the lack of drive or desire, but the act of building a system that works well enough to give you time to think about the next problem you want to solve. I’ve heard this described many times and love this description by Benoit Pimpaud.
2/ Practical Steps to Improve PLG - the team at UserOnboard creates excellent product-led growth teardowns like this one of SavvyCal. What I like best about this example are the detailed observations the team makes about copy, calls to action, and the placement of next actions for users. I learn something valuable from every teardown this team produces.
3/ Which convenience stores are located near each other? - This analysis of Japanese convenience store locations demonstrates the use of a basic data set and how to use this information for visualization or the prospective location of a new store. Why should you care? This is an excellent example of using a Python library for Open Street Map to analyze location data. Maybe you want to use this to map customers, activity data for consumers, or just do some deeper analysis for your next blog post. This is a cool trick to put in a Jupyter notebook.
What to do next
Hit reply if you’ve got links to share, data stories, or want to say hello.
Want more essays? Read on Data Operations or other writings at gregmeyer.com.
Want to book a discovery call to talk about how we can work together?
The next big thing always starts out being dismissed as a “toy.” - Chris Dixon